The SMART technologies that are being developed for on-farm disease diagnosis, and disease and water/milk quality monitoring can be supported with Big Data analytics for discovering new knowledge. In this research, the data that will be generated within the system infrastructure will be utilized using machine learning approaches. First, after determining baseline disease incidence and milk quality, spatial and temporal deviations from this baseline are determined. Deviations are investigated to define the threshold at which an early warning should be generated. Second, data from milk samples are used to train machine learning algorithms for the prediction of deviations in milk composition and mastitis. Third, the same is done for water quality monitoring, where the nutritional status of the pond and activity of the fish is monitored, and early warning systems are installed that identify under- or over-nutrification or deviant fish behavioural patterns. Fourth, the robustness of different types of ponds (e.g. shrimp versus fish, nutritious ponds versus regular ponds) against temporal and spatial deviations is analysed, making it possible to define the best system under different circumstances.
Two PhD students will work on PhD 9. The first PhD project (PhD 9a) focuses on the epidemiology and economic impact of Bovine Viral Disease Virus (BVDV) in Indonesia. The objective of the project is to investigate the dynamics of the disease and to assess its impact on small-scale dairy farming. The project will employ a longitudinal cohort study and machine learning approach to identify and analyze the risk factors associated with BVDV. Furthermore, bio-economical modeling will be used to estimate the economic impact of the disease. The results of the project will provide valuable inputs for farmers, cooperatives, and animal health stakeholders to design and implement effective disease control programs in dairy farming. Okta Wismandanu will work on the first part of PhD 9 (i.e. PhD 9a).
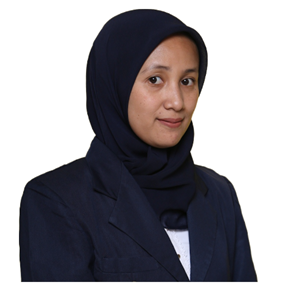
Okta Wismandanu
Contact: okta.wismandanu@wur.nl
This project is supervised by: Prof YH (Ynte) Schukken, Prof. Henk Hogeveen, Dr. Bart van den Borne
The second PhD project (PhD9b) focuses on a sensor-based automatic dairy cattle health monitoring decision support system. The objectives of the research project are to develop an automatic and reliable detection system for farmers’ decision support system on dairy cattle-specific diseases, i.e., metabolic diseases like ketosis. The sensor node transmitted information about the cattle’s physical condition. All the sensors are interfaced with the controller and a communication module that is capable of transmitting data. The sensor and computer vision nodes will send the data to the preparation module. The data preparation module is equipped with a pre-trained machine learning (ML) model, where it analyzes the data from all the nodes and provides information from them. The data from all nodes is combined and transmitted to analyze the condition of the cattle. The algorithm needs to be trained on a large set of training samples of healthy and diseased cows at various farms. In the event of an abnormality in cattle, it immediately transmits the information to the farmer or user on the cloud server. It provides health diagnosis and decision support for cattle treatment and disease prevention. Ultimately, a decision-support system that provides a diagnosis of the disease as well as a suggestion for a treatment will be developed for use on the users/farmers’ side. Disease prevention can be performed more effectively if there is adequate data and information. A farmer’s decision support system based on deep learning and mobile computing will allow farmers to acquire information to improve their productivity. Muhammad Ikhsan Sani will work on the second part of PhD 9 (PhD 9b).
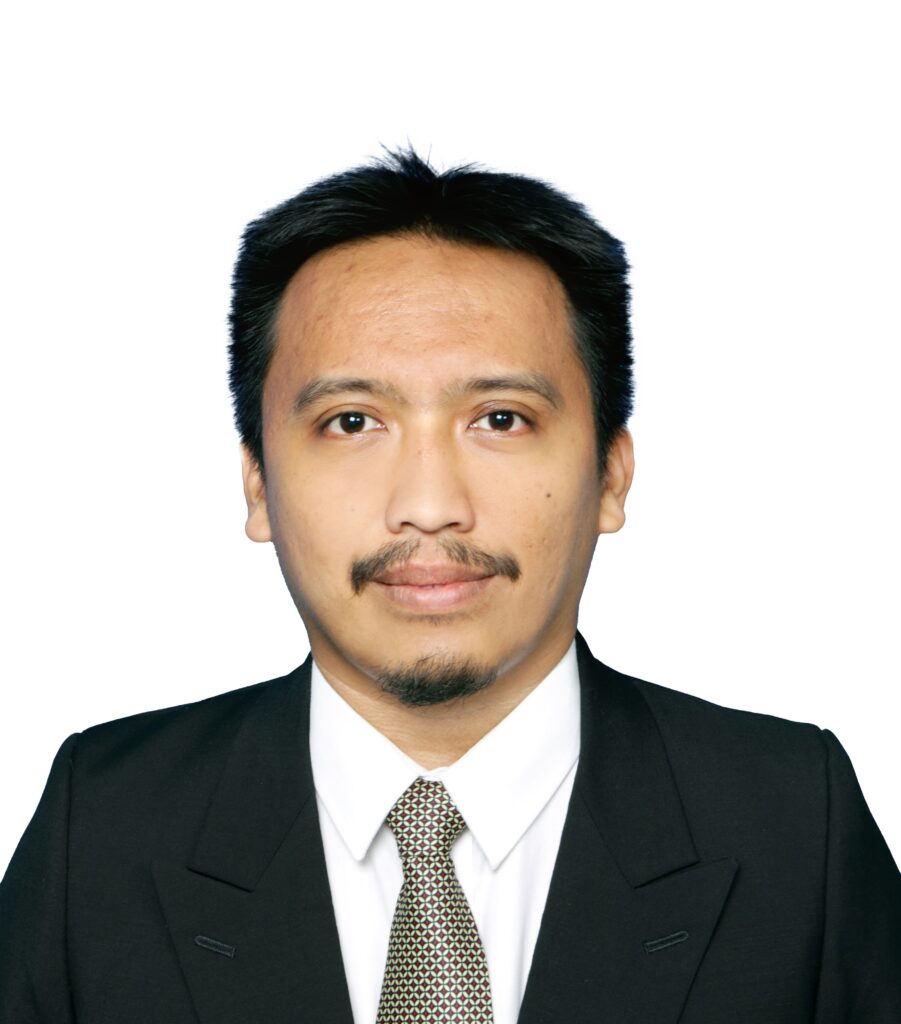
Muhammad Ikhsan Sani
Contact: m.ikhsan.sani@tass.telkomuniversity.ac.id
This project is supervised by: Prof. Henk Hogeveen, Dr. Mariska van der Voort